Working group management
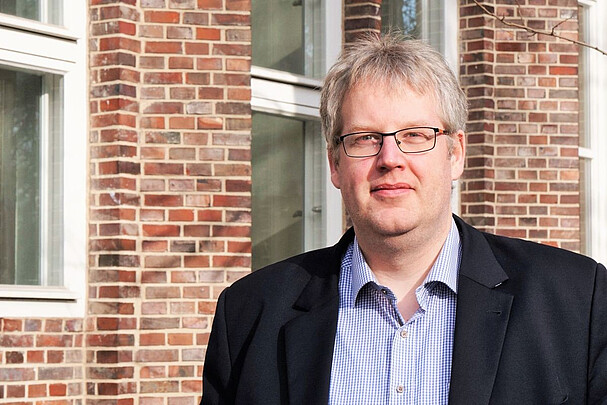
Prof. Dr.-Ing. Ingo Neumann
Executive Director
Phone
Address
Nienburger Straße 1-4
30167 Hannover
30167 Hannover
Building
Room
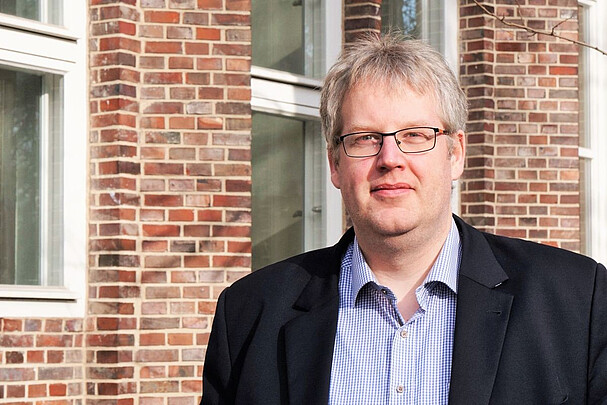
Prof. Dr.-Ing. Ingo Neumann
Executive Director
Working Group Management
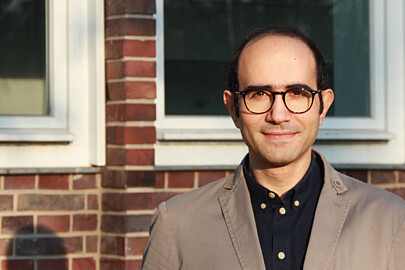
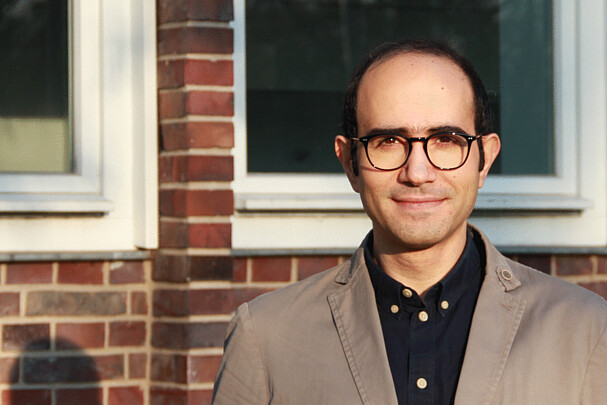
Dr.-Ing. Mohammad Omidalizarandi
Research Staff
Phone
Fax
Address
Nienburger Straße 1-4
30167 Hannover
30167 Hannover
Building
Room
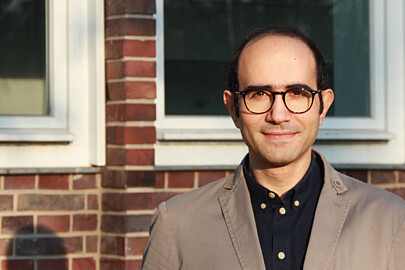
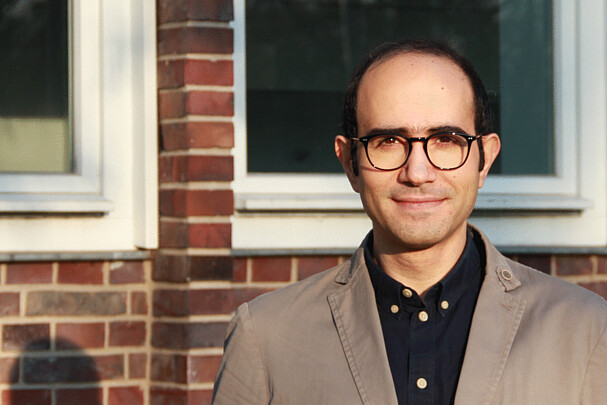
Dr.-Ing. Mohammad Omidalizarandi
Research Staff
Members of the working group
Current projects of the working group
-
Deformation analysis based on terrestrial laser scanner measurements (TLS-Defo, FOR 5455): Uncertainty of the surface approximationGeodetic deformation analysis involves the statistical analysis of geometric changes in two or more states. To exploit the full potential of established surface-based measurement techniques, such as terrestrial laser scanning (TLS), continuous local and global modelling of the monitored surface is required. The project ‘Uncertainty of Surface Approximation’ focuses on the investigation of the interaction between measurement and model uncertainties in the context of surface model selection. These components are closely related, since the amount of model uncertainty is directly influenced by the interaction between the complexity of the measured object, such as roughness and sharp edges, and the spatial density of measurement points over the object. To address this, the project differentiates between three subtopics: TLS uncertainty budget, model uncertainty and the application of fractal geometry as a methodological tool to achieve the primary project goal.Led by: Ingo Neumann, Mohammad OmidalizarandiTeam:Year: 2023Funding: DFGDuration: 10/23 – 09/27
-
port_AI – A fully digital twin for port structures using IoT, 5G, BIM, AR and AI methods to establish smart building lifecycle managementThe requirements for the safety and reliability of infrastructure management of infrastructure in the area of sea and inland ports are constantly increasing due to the growing globalisation of trade. The creation of a smart infrastructure should solve various challenges in the management of existing port infrastructure in this project. Digitisation and the use of AI processes are also included in this project under the term smart infrastructure. Only a thoroughly digital management of port infrastructure enables the economical use of resources, forward-looking maintenance, and early and comprehensive damage detection and assessment. This can lead to significant cost savings.Led by: Ingo Neumann, Hamza Alkhatib, Mohammad OmidalizarandiTeam:Year: 2021Funding: Funding programme for innovative port technologies (IHATEC) supported by the Federal Ministry of Transport and Digital Infrastructure (BMVI)Duration: 12/2021 – 02/2025